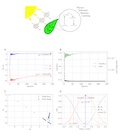
Discovering nonlinear resonances through physics-informed machine learning
For an ensemble of nonlinear systems that model, for instance, molecules or photonic systems, we propose a method that finds efficiently the configuration that has prescribed transfer properties. Specifically, we use physics-informed machine learning (PIML) techniques to find the parameters for the efficient transfer of an electron (or photon) to a targeted state in a nonlinear dimer. We create a machine learning model containing two variables, χD and χA, representing the nonlinear terms in the donor and acceptor target system states. We then introduce a data-free physics-informed loss function as 1.0 − Pj, where Pj is the probability, the electron being in the targeted state, j. By minimizing the loss function, we maximize the occupation probability to the targeted state. The method recovers known results in the targeted energy transfer (TET) model, and it is then applied to a more complex system with an additional intermediate state. In this trimer configuration, the PIML approach discovers desired resonant paths from the donor to acceptor units. The proposed PIML method is general and may be used in the chemical design of molecular complexes or engineering design of quantum or photonic systems.
Discovering nonlinear resonances through physics-informed machine learning.